Uncovering the grain of truth
- Space4Good
- Jun 27, 2022
- 5 min read
How CropLens uses remote sensing and geographic information system technology to drive pest control in smart rice farming
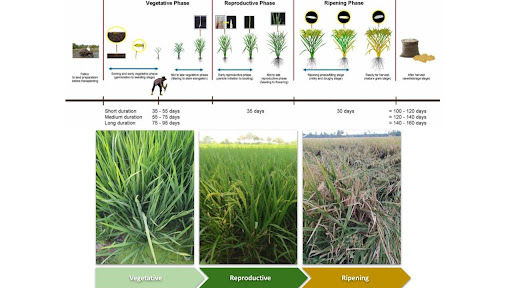
Figure 1: Paddy rice growth stages and duration
Partnering with like-minded entities to build damage-resilient crops
Space4Good, in collaboration with Cropin, is working on ‘CropLens’, a prototype early warning model for pests and diseases in a transplanted rice paddy that utilizes in-situ field observations, satellite data, and meteorological data together with AI. Bringing diverse experiences and skillsets to the table, the CropLens project has won the European funding via the Globalstars India funding mechanism as well as earned a EUREKA label supported by theNetherlands Enterprise Agency (RVO).
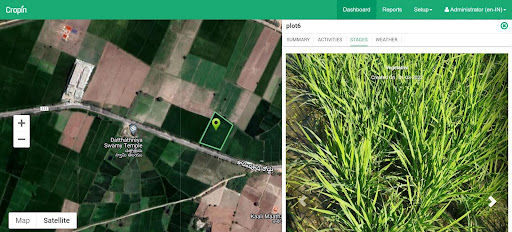
Figure 2: Field data visualization on the Smart farm application
Addressing challenges in rice farming using geospatial data analytics and Artificial Intelligence (AI)
According to estimates, farmers lose an average of 37% per annum of rice produce to pests and diseases, leading to substantial income losses. Various challenges are encountered by the farmers, such as the inability to:
Predict the occurrence and severity of pests and disease spread
Tackle the heterogeneous and fragmented characteristics of pest/disease infestation
Make conventional field scouting methods inexpensive, easy, and quick
Engage in early detection of pests and disease
Drive an impactful and cost-effective mitigation process, which can considerably reduce the need for harmful pesticides and water contamination
As such, there is an emerging need to leverage agri-tech platforms of remote sensing (RS) & Geographic Information Systems (GIS), and AI applications for pest detection and disease mitigation.
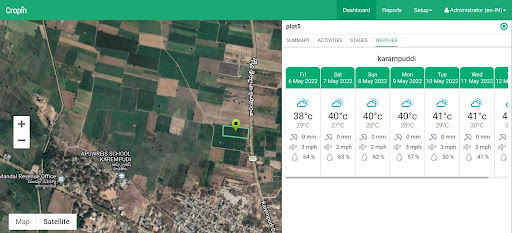
Figure 3: Weather data visualization on the Smart farm application
The CropLens pilot study: Reinventing–and restoring–the rice agroecosystem with space technology at its core
For the prototype pilot, the crop data is being collected from seven districts (West Godavari, East Godavari, Nellore, Guntur, Krishna, Prakasam, and Chittoor) in the State of Andhra Pradesh, India, and with a sample size of 500 farmers in each season (Kharif and Rabi). Farmers are selected using a random sampling method.
Objective #1: CropLens’ crop classification model will help in the identification of paddy fields.
End-goal: The CropLens model can:
Enable decision-makers, scientists, and also farmers to monitor and manage the paddy areas in a timely and sustainable manner.
Paddy rice mapping is crucial for agricultural mitigation and food security as they allow for quantification of paddy rice production and subsequent pest and disease modelling.
Objective #2: CropLens’ spatial hotspot maps will generate reports on the distribution and severity of predicted pest and disease infestation.
End-goal: The CropLens model can:
Empower farmers to make an informed decision and take proactive actions in preventing rice crop damage with real-time data at hand relating to pest and disease infestation
Objective #3: A data processing pipeline and a prototype of the web interface will enable the end-user to track crop health.
End-goal: The CropLens model can:
Enable the end-user, a.k.a farmers and land managers, to track crop health and prevent undue loss
Objective #4: Enable farmers to visualize crop risk
End-goal: The CropLens model can:
Give a clear picture of crop risk using remote sensing (RS) & Geographic Information systems (GIS), and artificial intelligence (AI) technologies
Objective #5: To provide insights and advisories to rice farmers in selected study areas on pest infestations and diseases
End-goals: The CropLens model can:
Detect early warnings of pest infestations and diseases in transplanted rice
Offer real-time pest and disease (P&D) alerts and advisories to farmers for timely action and crop protection
Understanding how the tech works at the ground level (and the space level)
CropLens comes with in-built Remote Sensing (RS) & Geographic Information System (GIS) technologies, making it an excellent tool for identifying pest and disease infestation remotely.
Here’s how the tool works:
Step 1: Using time-series satellite images, CropLens identifies the paddy-grown areas in the region.
↓
Step 2: CropLens identifies specific signatures of the likelihood of pest/disease infestation.
↓
Step 3: The tool augments this information with the weather data.
↓
Step 4: Improves modelling accuracy against certain weather conditions that favour infestation and facilitate spread.
The takeaway: CropLens can emerge as the key to solving common challenges of pest infestation in rice farming.
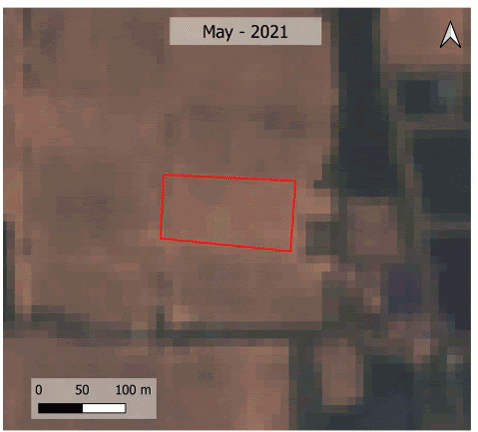
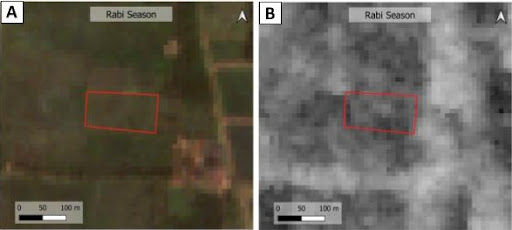
Figure 4: Visualization of a rice paddy field on a time series Sentinel-2 data during the growing season of 2021-22
The expected outcomes of using space-tech for productive smart rice farming using CropLens:
Crop classification maps for classifying and identifying the acreage of different crops in the region
Spatial hotspot maps portraying the distribution and severity of predicted pest and disease infestation
A data processing pipeline and a prototype of the web interface will help farmers and land managers to:
Keep track of crop health
Understand crop risk
Receive insights and advisories on how to proceed
The 360-degree proposed benefits of satellite data for one of the biggest commodities in the world, rice
CropLens is a comprehensive early warning system that can offer synoptic coverage in fragmented agricultural landscapes. With this effort, CropLens envisages helping small landholding farmers to:
Enhance crop productivity and boost their income
Employ sustainable agricultural practices
Use satellite remote sensing data as an effective replacement for ground surveys
Save on manual labour, time, and costs
After completing this study and developing a successful pest and disease forecast model, it is predicted that smallholder rice farmers will enjoy a –
20% increase in productivity driven by yield improvement
15% reduction in fungicide expenses
The road ahead: Strengthening–and advancing–smart farming with tech-powered data and insights
CropLens will be scaled to multiple crop types and agricultural landscapes as the momentum grows and the training potential increases. Furthermore, there is immense potential for institutions and stakeholders (like insurance companies) to contribute to improved risk management—an integral component that is lacking within the scope of this project.
The inclusion of these entities will help with improved dissemination of the pest and disease risk estimates and advisories with the early adopters such as farmers, supply chain managers, governmental entities, and the entire ecosystem at large.

About Cropin: A pioneer in agri-tech
Cropin, a pioneer in the Agri-tech space, is building the first and most comprehensive global Intelligent Agriculture Cloud. Chopin's platform enables various stakeholders in the agri-ecosystem to leverage digitization and AI at scale, to make decisions that increase efficiency, scale production, and strengthen sustainability.
Cropin has worked with 250+ customers and has digitized 16 million acres of farmland, improving the livelihoods of more than 7 million farmers. It has built the world’s largest farming data insights over a decade, spearheading a global ‘Ag-intelligence’ movement with a knowledge graph of 488 crops and 10,000 crop varieties in 56 countries. With its AI/ML platform tailor-made for the agriculture ecosystem, Cropin has computed 0.2 billion acres of farmland in 12 countries, covering 24 major commodities.
The original case study can be found on the CropLens website here.
Would you like more information or are you interested in collaborating with Space4Good? Visit our website or contact us via hello@space4good.com.
Коментарі